DeepNash learns to play Stratego from scratch by combining sport idea and model-free deep RL
Sport-playing synthetic intelligence (AI) programs have superior to a brand new frontier. Stratego, the basic board sport that’s extra advanced than chess and Go, and craftier than poker, has now been mastered. Published in Sciencewe current DeepNashan AI agent that discovered the sport from scratch to a human professional stage by taking part in in opposition to itself.
DeepNash makes use of a novel method, based mostly on sport idea and model-free deep reinforcement studying. Its play type converges to a Nash equilibrium, which suggests its play could be very exhausting for an opponent to use. So exhausting, the truth is, that DeepNash has reached an all-time top-three rating amongst human specialists on the world’s largest on-line Stratego platform, Gravon.
Board video games have traditionally been a measure of progress within the discipline of AI, permitting us to check how people and machines develop and execute methods in a managed setting. In contrast to chess and Go, Stratego is a sport of imperfect info: gamers can not instantly observe the identities of their opponent’s items.
This complexity has meant that different AI-based Stratego programs have struggled to get past newbie stage. It additionally signifies that a really profitable AI approach known as “game tree search”, beforehand used to grasp many video games of good info, shouldn’t be sufficiently scalable for Stratego. Because of this, DeepNash goes far past sport tree search altogether.
The worth of mastering Stratego goes past gaming. In pursuit of our mission of fixing intelligence to advance science and profit humanity, we have to construct superior AI programs that may function in advanced, real-world conditions with restricted info of different brokers and other people. Our paper exhibits how DeepNash might be utilized in conditions of uncertainty and efficiently steadiness outcomes to assist clear up advanced issues.
Attending to know Stratego
Stratego is a turn-based, capture-the-flag sport. It’s a sport of bluff and ways, of knowledge gathering and delicate manoeuvring. And it’s a zero-sum sport, so any achieve by one participant represents a lack of the identical magnitude for his or her opponent.
Stratego is difficult for AI, partly, as a result of it’s a sport of imperfect info. Each gamers begin by arranging their 40 taking part in items in no matter beginning formation they like, initially hidden from each other as the sport begins. Since each gamers do not have entry to the identical data, they should steadiness all potential outcomes when making a choice – offering a difficult benchmark for finding out strategic interactions. The kinds of items and their rankings are proven under.
Center: A potential beginning formation. Discover how the Flag is tucked away safely on the again, flanked by protecting Bombs. The 2 pale blue areas are “lakes” and are by no means entered.
Proper: A sport in play, displaying Blue’s Spy capturing Purple’s 10.
Info is tough received in Stratego. The identification of an opponent’s piece is often revealed solely when it meets the opposite participant on the battlefield. That is in stark distinction to video games of good info corresponding to chess or Go, during which the placement and identification of each piece is thought to each gamers.
The machine studying approaches that work so effectively on good info video games, corresponding to DeepMind’s AlphaZeroare usually not simply transferred to Stratego. The necessity to make selections with imperfect info, and the potential to bluff, makes Stratego extra akin to Texas maintain’em poker and requires a human-like capability as soon as famous by the American author Jack London: “Life is not always a matter of holding good cards, but sometimes, playing a poor hand well.”
The AI methods that work so effectively in video games like Texas maintain’em don’t switch to Stratego, nonetheless, due to the sheer size of the sport – usually tons of of strikes earlier than a participant wins. Reasoning in Stratego have to be executed over numerous sequential actions with no apparent perception into how every motion contributes to the ultimate end result.
Lastly, the variety of potential sport states (expressed as “game tree complexity”) is off the chart in contrast with chess, Go and poker, making it extremely tough to resolve. That is what excited us about Stratego, and why it has represented a decades-long problem to the AI neighborhood.
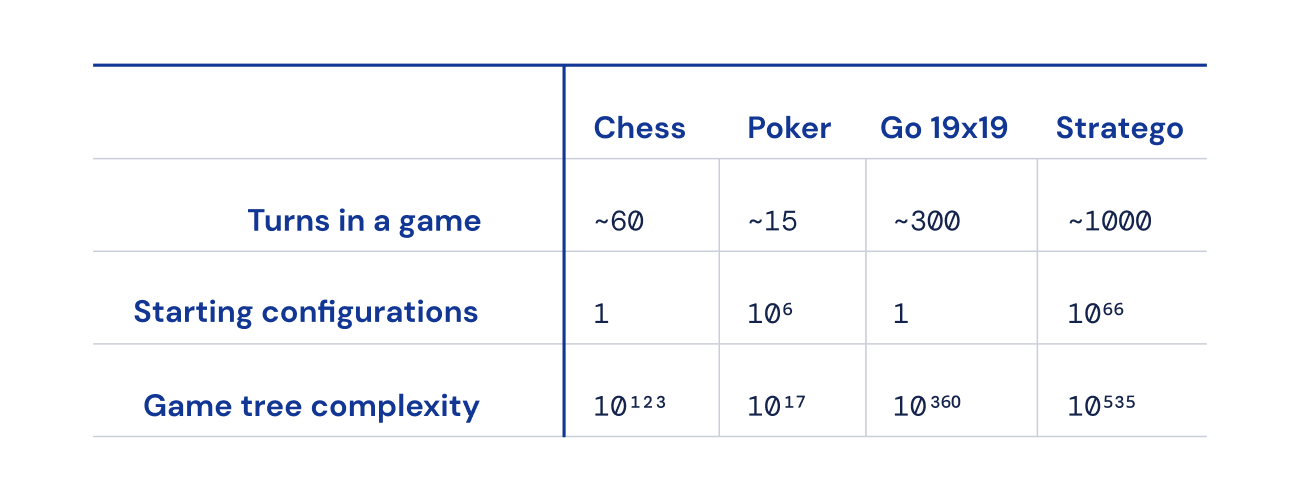
In search of an equilibrium
DeepNash employs a novel method based mostly on a mixture of sport idea and model-free deep reinforcement studying. “Model-free” means DeepNash shouldn’t be making an attempt to explicitly mannequin its opponent’s non-public game-state in the course of the sport. Within the early levels of the sport specifically, when DeepNash is aware of little about its opponent’s items, such modelling could be ineffective, if not unimaginable.
And since the sport tree complexity of Stratego is so huge, DeepNash can not make use of a stalwart method of AI-based gaming – Monte Carlo tree search. Tree search has been a key ingredient of many landmark achievements in AI for much less advanced board video games, and poker.
As an alternative, DeepNash is powered by a brand new game-theoretic algorithmic concept that we’re calling Regularised Nash Dynamics (R-NaD). Working at an unparalleled scale, R-NaD steers DeepNash’s studying behaviour in direction of what’s often called a Nash equilibrium (dive into the technical particulars in our paper).
Sport-playing behaviour that leads to a Nash equilibrium is unexploitable over time. If an individual or machine performed completely unexploitable Stratego, the worst win fee they may obtain could be 50%, and provided that going through a equally good opponent.
In matches in opposition to the most effective Stratego bots – together with a number of winners of the Pc Stratego World Championship – DeepNash’s win fee topped 97%, and was incessantly 100%. In opposition to the highest professional human gamers on the Gravon video games platform, DeepNash achieved a win fee of 84%, incomes it an all-time top-three rating.
Count on the surprising
To realize these outcomes, DeepNash demonstrated some outstanding behaviours each throughout its preliminary piece-deployment part and within the gameplay part. To develop into exhausting to use, DeepNash developed an unpredictable technique. This implies creating preliminary deployments diversified sufficient to forestall its opponent recognizing patterns over a collection of video games. And in the course of the sport part, DeepNash randomises between seemingly equal actions to forestall exploitable tendencies.
Stratego gamers attempt to be unpredictable, so there’s worth in retaining info hidden. DeepNash demonstrates the way it values info in fairly putting methods. Within the instance under, in opposition to a human participant, DeepNash (blue) sacrificed, amongst different items, a 7 (Main) and an 8 (Colonel) early within the sport and consequently was in a position to find the opponent’s 10 (Marshal), 9 (Basic), an 8 and two 7’s.
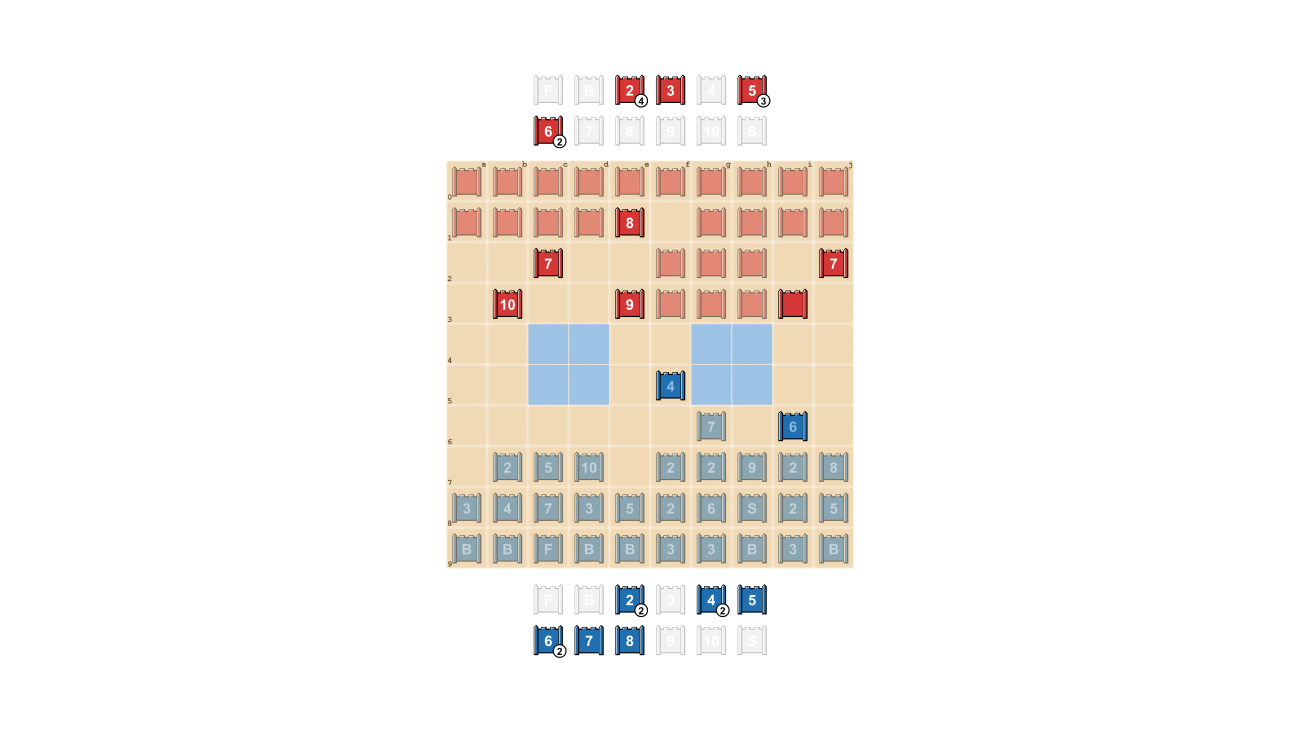
These efforts left DeepNash at a big materials drawback; it misplaced a 7 and an 8 whereas its human opponent preserved all their items ranked 7 and above. However, having stable intel on its opponent’s high brass, DeepNash evaluated its profitable probabilities at 70% – and it received.
The artwork of the bluff
As in poker, a great Stratego participant should typically symbolize energy, even when weak. DeepNash discovered quite a lot of such bluffing ways. Within the instance under, DeepNash makes use of a 2 (a weak Scout, unknown to its opponent) as if it have been a high-ranking piece, pursuing its opponent’s recognized 8. The human opponent decides the pursuer is more than likely a ten, and so makes an attempt to lure it into an ambush by their Spy. This tactic by DeepNash, risking solely a minor piece, succeeds in flushing out and eliminating its opponent’s Spy, a essential piece.
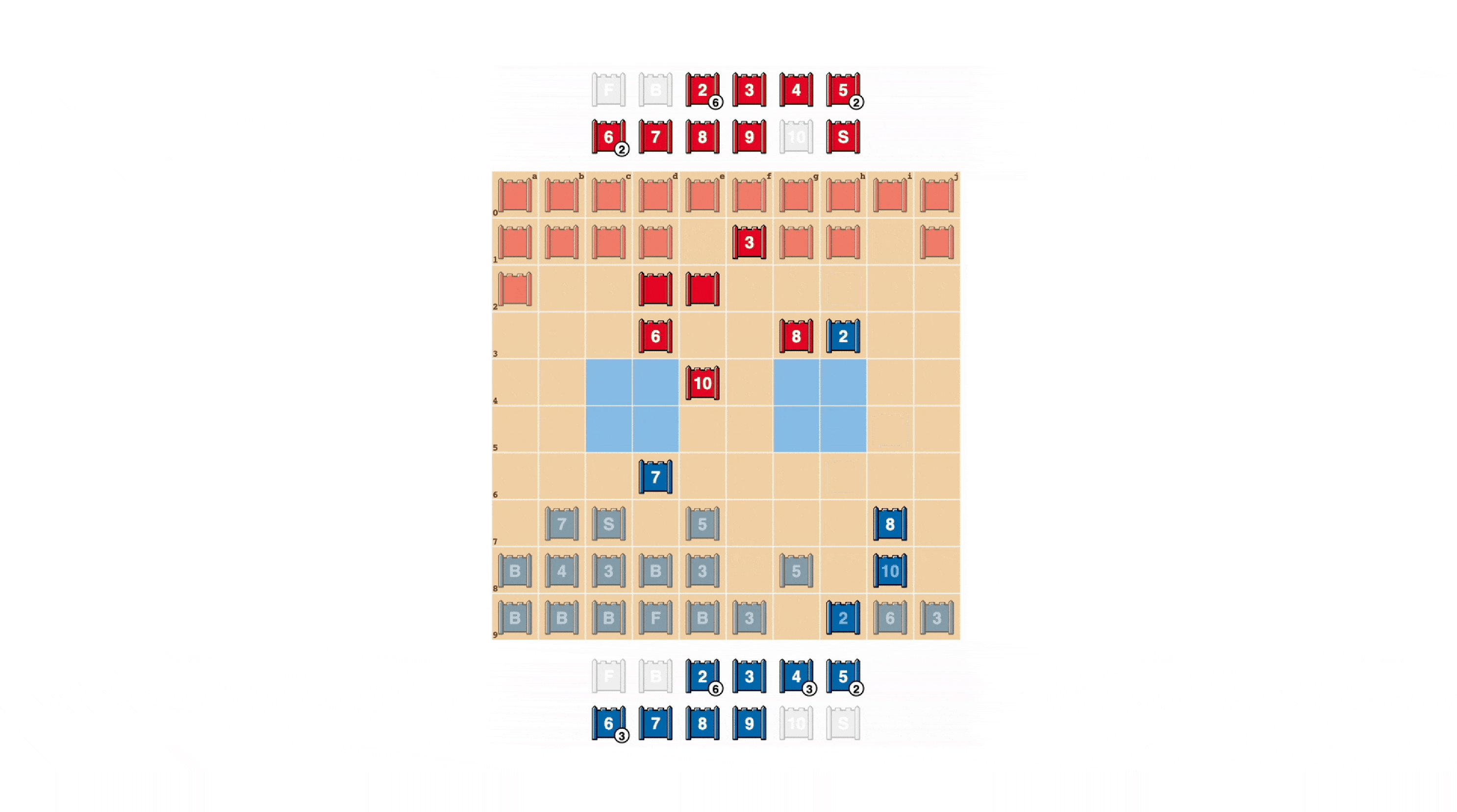
See extra by watching these 4 movies of full-length video games performed by DeepNash in opposition to (anonymised) human specialists: Game 1, Game 2, Game 3, Game 4.
“The level of play of DeepNash surprised me. I had never heard of an artificial Stratego player that came close to the level needed to win a match against an experienced human player. But after playing against DeepNash myself, I wasn’t surprised by the top-3 ranking it later achieved on the Gravon platform. I expect it would do very well if allowed to participate in the human World Championships.”
– Vincent de Boer, paper co-author and former Stratego World Champion
Future instructions
Whereas we developed DeepNash for the extremely outlined world of Stratego, our novel R-NaD methodology might be instantly utilized to different two-player zero-sum video games of each good or imperfect info. R-NaD has the potential to generalise far past two-player gaming settings to handle large-scale real-world issues, which are sometimes characterised by imperfect info and astronomical state areas.
We additionally hope R-NaD can assist unlock new functions of AI in domains that characteristic numerous human or AI members with completely different objectives that may not have details about the intention of others or what’s occurring of their setting, corresponding to within the large-scale optimisation of visitors administration to scale back driver journey occasions and the related car emissions.
In making a generalisable AI system that’s strong within the face of uncertainty, we hope to deliver the problem-solving capabilities of AI additional into our inherently unpredictable world.
Study extra about DeepNash by studying our paper in Science.
For researchers enthusiastic about giving R-NaD a attempt or working with our newly proposed methodology, we’ve open-sourced our code.
Author:
Date: 2022-11-30 19:00:00